Category Archives: Tools
Films as an Educational Tool and Digital Story Telling
I am not going to hide the fact that I have used film in my work as a social studies teacher so I will be honest and say I have used film as a means to show my students how things would have looked and sounded like in the past. I have also used film as a means of giving character and humanity to historical figures and participants. Film is also a great way to put historical figures and events in context. Eighth grade students have difficulty understanding/conceptualizing the past because they have lacked an exposure to it. The cinema is not as popular as it once was and youth culture is more fragmented than unified when it comes to popular media due to cellphones and social media. After reading and learning what I have, I am planning to use film a lot more than I originally have. The line of historical inquiry I will use will rely on a similar line of questioning associated with photo analysis. Who is the creator? When was the film created? When and where does the film take place? What historical event(s)/people are depicted? And, so on. However, a different line of questioning will be given to students based on specifics associated with the given film.
I have used clips from films like Last of the Mohicans (1992), John Adams (HBO Mini series, 2008) and Gone with the Wind (1939) to give context to historical moments, figures, and participants as well as to give reasoning for the causes and effects behind historical events. I am on the lookout for other films and television programs that are related to historical events from the 1700s to 1870s.
Learners can benefit from digital story telling because it allows them to conduct a more in depth study of historical content. Digital story telling can serve as a step up from traditional methods like essay writing by enabling students to interact with historical research in an innovative way. Students are more likely to experiment and take risks with new methodologies and multimedia resources that re-represent historical data and information. These projects can then be shared and revised more easily with collaborators and audiences.
Podcasts from the Perspectives of the Digital Humanities
Podcasts are transformative in the way they can enhance digital humanities research that would otherwise be printed in academic papers. Podcasts transform academic scholarly tradition by taking advantage of the theatrical nature of podcasts. Dan Cohen, Vice Provost, Dean and Professor of Northeastern University, praises podcasts for their ability to genre-build as well as their “unparalleled ability to convey the reality of an academic work, and inculcate appreciation of it.” Essentially, podcast elements like sound design, audio editing, voice acting and narration, make scholarly research more compelling for a general audience. Podcasts such as NPR’s Throughline, Consolation Prize, and Ben Franklin’s World demonstrate the effectiveness of using audio design, sound mixing and voice acting in podcasting. They utilize hosts, experts and voice actors and sound effects to help drive the narratives being discussed and make the content more engaging for the listener. These podcast elements enable the listener to become more acquainted and engrossed with the narrative.
Similarly to the radio shows of the mid-twentieth century, podcasts communicate a story that is compelling to the listener. Fortunately, according to Pew Research data, a majority of podcast listeners engage with podcasts for entertainment, learning and diversion. In other words, educational podcasts that share scholarly research are only growing in popularity with the general public.
For the most part, podcasts differ from other tools in that they are more theatrical in the conveyance of academic research. Podcast elements such as sound design and voice acting make text based research more interesting to a general viewer. Podcasts also enable research to become more of a conversation to be had with multiple individuals such as the host of the podcast and the experts. The host helps guide the discussion by providing historical context and guiding questions that will help the listener grasp the narrative that is being told by the expert.
With all that is said with the existing differences, podcasts share a similar role with other data tools residing within the digital humanities. Data tools like Voyant, kepler.gl and Palladio share a commonality with podcasts as they all serve as re-representations of data that can be comprehended and interpreted for people to reach new conclusions about the human experience. While we may not typically conduct an analysis of podcasts, we can use tools like Perusall to annotate what is spoken or done in podcasts to keep track of source and citation information. This can then assist users in pursuing further research on a given topic. AI such as ChatGTP and crowdsourced knowledge projects like Wikipedia also lend themselves to the conversational and discussion style utilized by podcasts. Crowdsourcing projects just like podcasts also engage public audiences with scholarly research in a non-traditional fashion.
According to scholar Trevor Owens, the digital humanities “are at its best when it embraces the digital not simply as a means to the traditional ends of scholarship, but when it transforms the nature of what humanists do.” Fortunately, podcasts fit this understanding as they can drastically change the way in which scholarly research is shared and explored. Scholarly research no longer has to depend on its publication in academic papers but can now be shared in a digital format that can be listened to and shared with everyone to further the digital humanities.
What Can Digital Humanities Do With Crowdsourcing?
Within the digital humanities, crowdsourcing is the methodology of recruiting volunteers from the general public to engage in cultural research via digital platforms. The purpose of it is to encourage volunteers to work with researchers, archivists and academics to gather information that serves the greater cultural heritage community by gaining and spreading knowledge. Crowdsourcing is best suited for digital projects that involve transcription. Crowdsourcing transcription can be a successful endeavor as long as its digital interface is easy to understand and accessible.
The Library of Congress’ By the People initiative is a perfect example of a crowdsourcing project that is easy to understand and accessible. Users do not have to create an account to begin their transcription services. The project is up front about which transcription projects are in progress and/or completed. The project also provides easy to understand parameters for transcription. Instructions are provided for how users can get started, transcribe, review and tag. A History Hub discussion forum is also provided for users in case they come across additional issues with the transcription process. Additionally, it is helpful that the project staffs a room of professionals who can monitor the platform.
The digital humanities can benefit from crowdsourcing in that it can transform the way cultural heritage artifacts are taught and learned about. For the most part, crowdsourcing allows more people to access artifacts and institutions. Plus, first hand experience with transcription enables volunteers to pursue further knowledge or engagement with a specific subject matter.
How to Read Crowdsourced Knowledge
Using Wikipedia:
Wikipedia offers its users a unique opportunity to explore the talk and history pages of its articles. The exploration of the talk and view history pages gives users transparency on the creation of Wikipedia articles. Users can ultimately gain insight on the dialogue contributors have on article creation as well as the revising and editing process of Wikipedia pages.

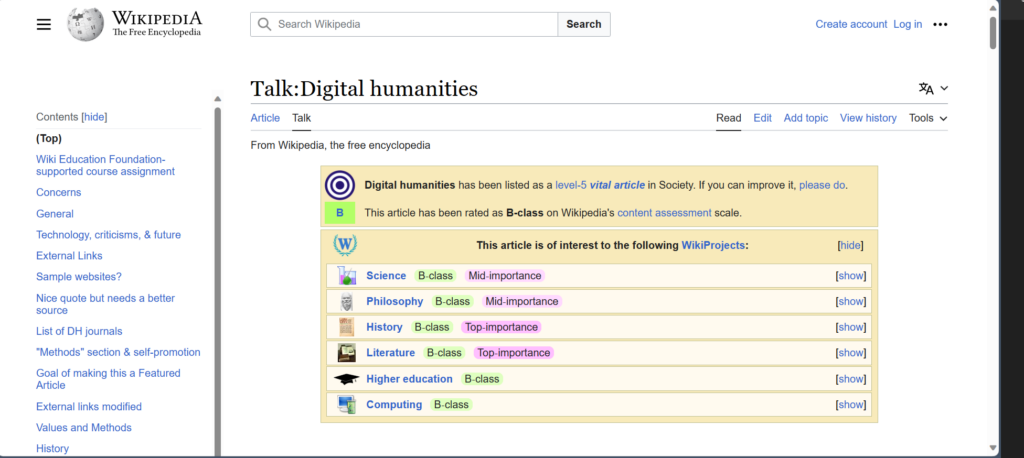
I utilized Wikipedia’s talk and history features in my exploration of Wikipedia’s digital humanities’ article. The talk feature allowed me to see the discussions contributors had over the content that was written. There were apparent issues related to the article’s organization. The article also had numerous contributors who engaged in a dialogue on making improvements to the article’s quotations, external links, neutrality, definitions and so on. Users are also allowed to view the biographies of the contributors. For instance, Skirden and Hectorlopez17 were top contributors for the current digital humanities article. They were both associated with Wikipedia projects like Wikipedian in Residence and the Electronic Textual Cultures laboratory. If the information is public, users can see the identified credentials of the key contributors and their associations. In regard to the history, I could see that the article was first created on January 30, 2006 by contributor Elijahmeeks. Since 2006, the page has evolved to include more information related to the digital humanities such as its definition, history, cultural relevance, project purpose, values and methods, criticism, references and external links.

That said, Wikipedia is not entirely perfect. It is open source which means anyone can access its entries. But, its transparency with features like their talk and history pages enables users to see how ideas related to their articles have changed overtime; therefore, enabling a reliance on user judgment.
Using AI:
Just like Wikipedia, Artificial Intelligence programs are not without their own problems and possibilities. Programs like ChatGPT have weaknesses in citing the location of information they utilize in their response. ChatGPT also generates fictional data points when it does not have enough information to answer an unfamiliar question. ChatGPT also has the potential to oversimplify complex issues due to a lack of human input as it is an AI. However, these flaws can be improved with the implementation of human feedback and plug-in creation.

According to Jon Gertner, AI companies have made moves to correct AI misinformation by using reinforcement learning with human feedback while the Wikimedia Foundation has created a Wikipedia based plug-in to redirect ChatGPT to Wikipedia for further information on a subject matter. For the most part, AI has the potential to work with users to answer questions in a conversational format that can initiate deeper inquiries into various subject matters. Also, the proposed improvements for AI can lead users into carrying their own scholarly research once AI can provide the necessary citations.
Comparing Tools
Software tools like Voyant, kepler.gl, and Palladio can be used to create visual rerepresentations of research findings. The tools expand on existing computational methods and techniques. These existing computational methods include textmining, mapping and network analysis. Amongst other similarities, Voyant, kepler.gl, and Palladio can be used to demonstrate the relationships that exists within the research data. Users can upload their digitized research data into the given software to create visualizations of the data. The visualizations will then enable the data to be interpreted and analyzed. However, software tools vary in how they can visually enhance information and what digitization techniques can be used collaboratively.
Voyant is preferred when textmining data. Voyant enables users to upload links of textual information into data sets to be processed into a digital corpus. The digital corpus can then be modified into Voyant’s visual interactive tools like Cirrus, Reader, Trends, Summary and Contexts. For example, I filtered data from the WPA slave narratives to compare the frequency of words in various states. I was then able to conduct a textual analysis of distinctive words based on location. From these tools, users can conduct data analysis to determine relevant patterns and relationships.
Comparatively, kepler.gl is a digitized mapping tool. Users can utilize features like points, clusters and heat maps to enhance uploaded data. The assortment of maps enable the exploration of data point density to illuminate the significance of certain locations. Kepler.gl also offers features like category maps and timelines to demonstrate the changes and continuities that exist over time in a data set. Kepler.gl also enables data differentiation. I was able to use kepler.gl to differentiate the different jobs the enslaved (identified in the WPA slave narratives) were assigned. I was also able to use the timeline feature to see changes in the data overtime to examine the relationships between various locations and their significance in Alabama.
Palladio, a digital network graphing tool, can also be used to create visual interactives. Users can upload data that can be networked within the software. Palladio allows its users to modify the dimensions, layers, sources, targets, facets, nodes and links of uploaded data. With these modifications, users can evaluate the patterns and connections that exist in the data. In my usage of the WPA slave narratives, I was able to identify patterns in the data related to unknown ages of the interviewees by using the provided dimensions.
Clearly, all three software tools are useful in showing the relationships and patterns that exist in uploaded data. But, they differ in what computational methods they can be best utilized. Voyant is preferred for textmining while kepler.gl and Palladio are useful for data mapping. Despite the differences, these three software tools can be used in collaboration to provide various visual interactives that can be used to confirm or deny patterns and continuities in the data. The given software can also be used to draw support for historical interpretations by drawing on the quantitative and qualitative evidence presented in textmining and mapping.
Network Analysis with Palladio
What is a network graph?
A network graph is a digital diagraming tool that can be used to visually represent the relationships that exist within research data by expanding on current digital tools like OCR, text-mining and mapping. A network graph relies on digitized data, nodes and links to create visual interactives and representations of the given information. From these visualizations, researchers can interpret the patterns that exist in the information. Notably, researchers can use their research data in software like Palladio to create a deeper understanding for their subject matter as well as to determine the relevant connections and inquiries that exist within and from the data.
Wait? What is Palladio?
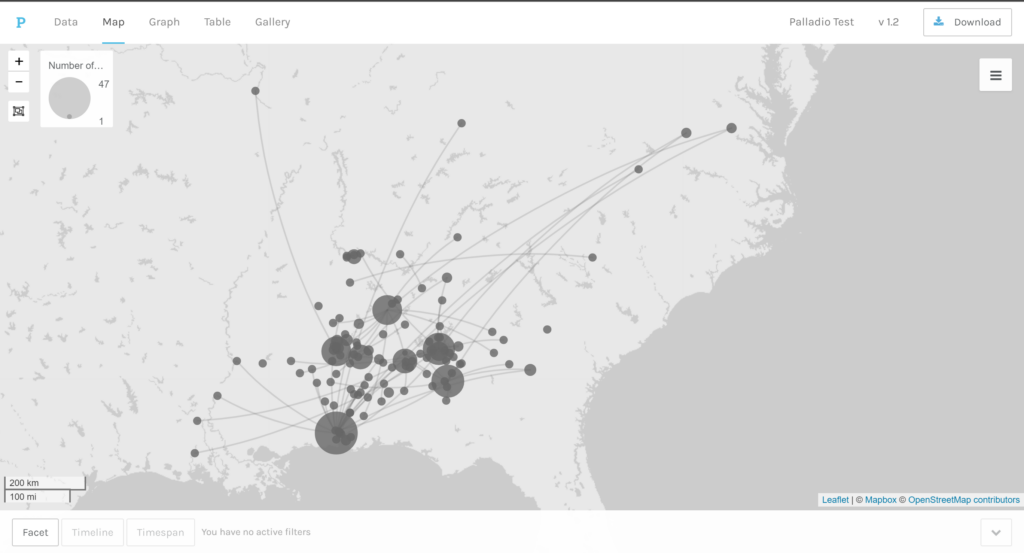
Palladio is a software tool that enables researchers to upload relevant research data from a .csv or spreadsheet that can be networked within the software. From there, Palladio can create visual interactives using nodes and links based on the dimensions, layers, sources, targets and facets set by the user. In my test run of the software, I uploaded data from the WPA slave narratives project. I was able to filter my network graphs based on my selected dimensions such as M/F (Male/Female), Type of Work, Interviewer, Topics, Age, When interviewed and so on. As a result, I was able to see the connections and patterns that existed within the data. For instance, I used the network graph to see that there was a large node associated with ages that were unknown to the interviewers. This led me to the conclusion that many of the former enslaved had no idea what their age was based on a lack of record keeping that occurred on plantations for the enslaved.
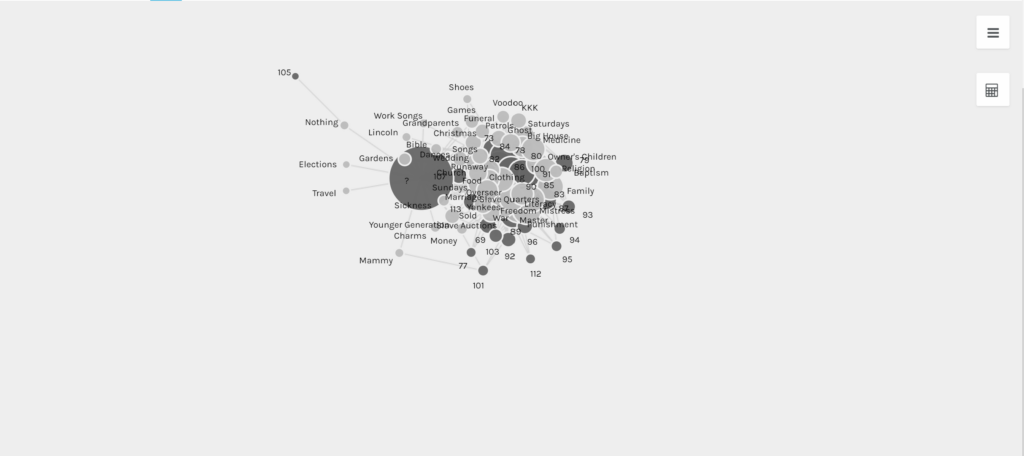
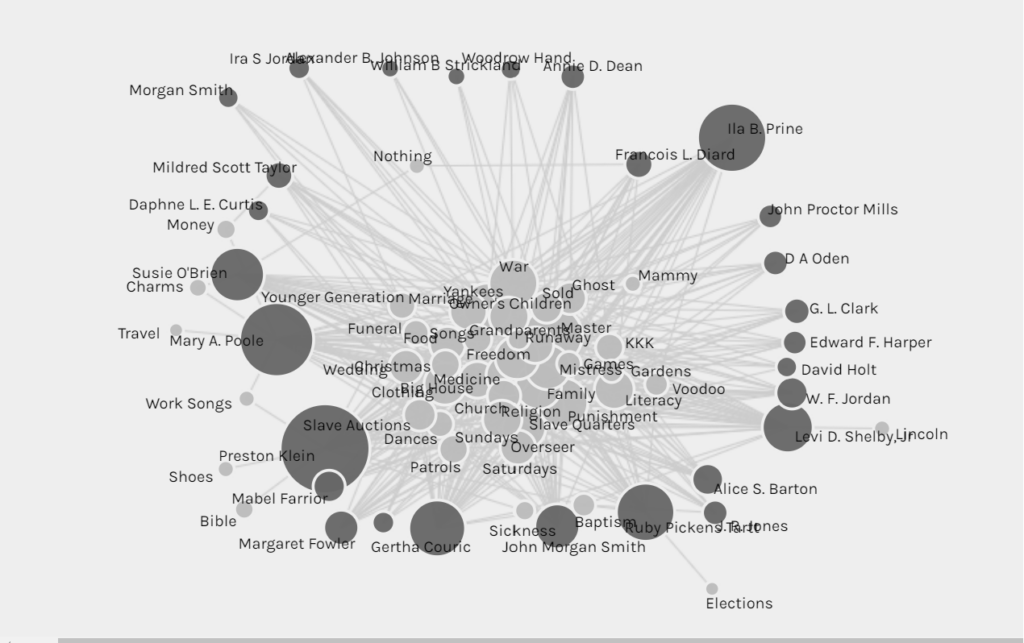
How have network graphs been used?
Network graphs have also allowed scholars to explore the connections and inquiries associated with the reprinting of texts in the nineteenth century (the Viral Texts Project), the correspondence of Enlightenment thinkers (Mapping the Republic of Letters) and the personal relationships of Jazz musicians (Linked Jazz). The Viral Texts Project demonstrates the culture of reprinting in the nineteenth century as well as the popularity of certain texts and themes in that period of time. Comparatively, the Mapping the Republic of Letters project allows scholars to see the transference of ideas that emerged with the correspondence and relationships of certain historical figures. Similarily, the Linked Jazz project diagrams the relationships of various Jazz musicians by linking documents and data from archives, libraries and museums. Understandably, network graphs enable scholars to learn new insights on the research they currently have.
Text Analysis with Voyant
Textmining allows you to use computer software to conduct an extensive quanitative analysis of text. Textmining is most useful in conducting an analysis of large corpora in a database or archive. Specifically, textmining can be used to search through text to quanitify how often a certain word appears in a digitized archive or material. Textmining tools can be used to examine patterns or trends in qualitiative data. Several projects that have utilized textmining include America’s Public Bible, Robots Reading Vogue and Signs40. In the case of America’s Public Bible, textmining was used to perform an analysis of biblical quotations in US newspapers to determine trends in biblical references at different historical periods. Robots Reading Vogue was designed to evaluate beauty trends over time while Signs40 used textmining to determine changes in Signs’ feminist scholarship. Trends related to certain words, topics and themes can then enable a researcher to draw certain conclusions on the historical time period of study.
Free text mining tools like Voyant can be used to conduct textual analysis. For instance, you can upload links of textual information to Voyant as a dataset that will then be processed into a digital corpus. From there, tools like Cirrus, Reader, Trends, Summary and Contexts can be used as visual interactives to find patterns or differences in the data. The dataset used for my exploration of Voyant was the WPA Slave Narratives.
I utilized all of Voyant’s given tools to compare the frequency of words in various states. Specifically, I compared the states of Alabama and Maryland to each other by seeing the distinctive words utilized in their interviews. For instance, Alabama used distinct words like didn’t (357), alabama (138), ain’t (213), don’t 182), ca’se (69), caze(104), couldn’t (103), i’s (93), i’se (92), dat’s (90). Maryland used distinct words like bowie site. (17), baltimore (64), ellicott(11), cockeysville (11), arundel (9), annapolis (9), md (11), maryland (60), rezin(7), manor (6). From this analysis, I was able to conclude that Alabama’s distinct words referred to their unique dialect while Maryland’s distinct words referred to Maryland African American communities. In using Voyant, I was able to see that while textmining is useful in searching through large sets of data-it is imperative that a person is working in conjuction with the software to get rid of unnesessary “stop” words and to make sense of the words that are repeated throughout to draw historical conclusions.
Why Metadata Matters
Metadata is “structured textual information that describes something about the creation, content or context of a digital resource” (Jisc, 2014). Metadata enables items to be discoverable in a database or archive due to the field information provided. Researchers, patrons and digital humanists can use the metadata provided in common fields like title, subject, description, creator, date, format, type and location to find artifacts and documents.The common fields present digital humanists with a source’s context and descriptive infromation. Context and description are the most essential parts of metadata as they help digital humanists understand “the physical and digital origin, structure, and technical makeup” of a resource (Carbajal and Caswell, 2021, pg.1108 ). From this, digital humanists can improve their own understanding of their research and appropriately utilize primary and secondary sources in the digital humanities.
Additionally, metadata serves a purpose in maintaining an archive’s material infrastructure which can then aid the digital humanities as a whole. Maintaining infrastructure through the preservation of physical and digital records will feed into the ever changing architectural, technological, social, epistemolgical, and ethical structures of an archive (Carbajal & Caswell, 2021). Consequently, digital archivists can fullfill the needs of their digital users by adopting improved metadata standards to extinguish problematic metadata. The continued maintenance of material infrastructure has the power to place checks on the political, historical, and cultural biases of the archivist or archive in order to better satisfy the needs of a diverse population of digital users.
Tropy and Omeka can assist digital humanities practitioners in research organization and collaboration. Trophy is a software tool and unified system that enables researchers to manage and describe the image files of archival materials. Archival photographs can be saved and viewed for future reference within Tropy. Specifically, common field information for resources can be saved within the software and organized into various projects and collections. Similarly, Omeka serves as a computer software that assists researchers in organizing their materials. Omeka also has interoperability which allows users to share Tropy’s archival metadata with a larger audience. For instance, I am now able to share my findings related to Service Guardian and Hamilton Beach cookware. Software like Tropy and Omeka enabled me to become more thorough with locating and writing the correct metadata. Since Omeka is a resource that can be shared with others, I wanted to make sure that I was using the correct subject, name, title, and name authorities for my kitchen collection. I also wanted to develop enough accurate background knowledge on my kitchen items to provide the correct contextual and descriptive information. Fortunately, Tropy and Omeka enable users to follow the best practices in maintaining metadata by offering archival organization and information sharing.